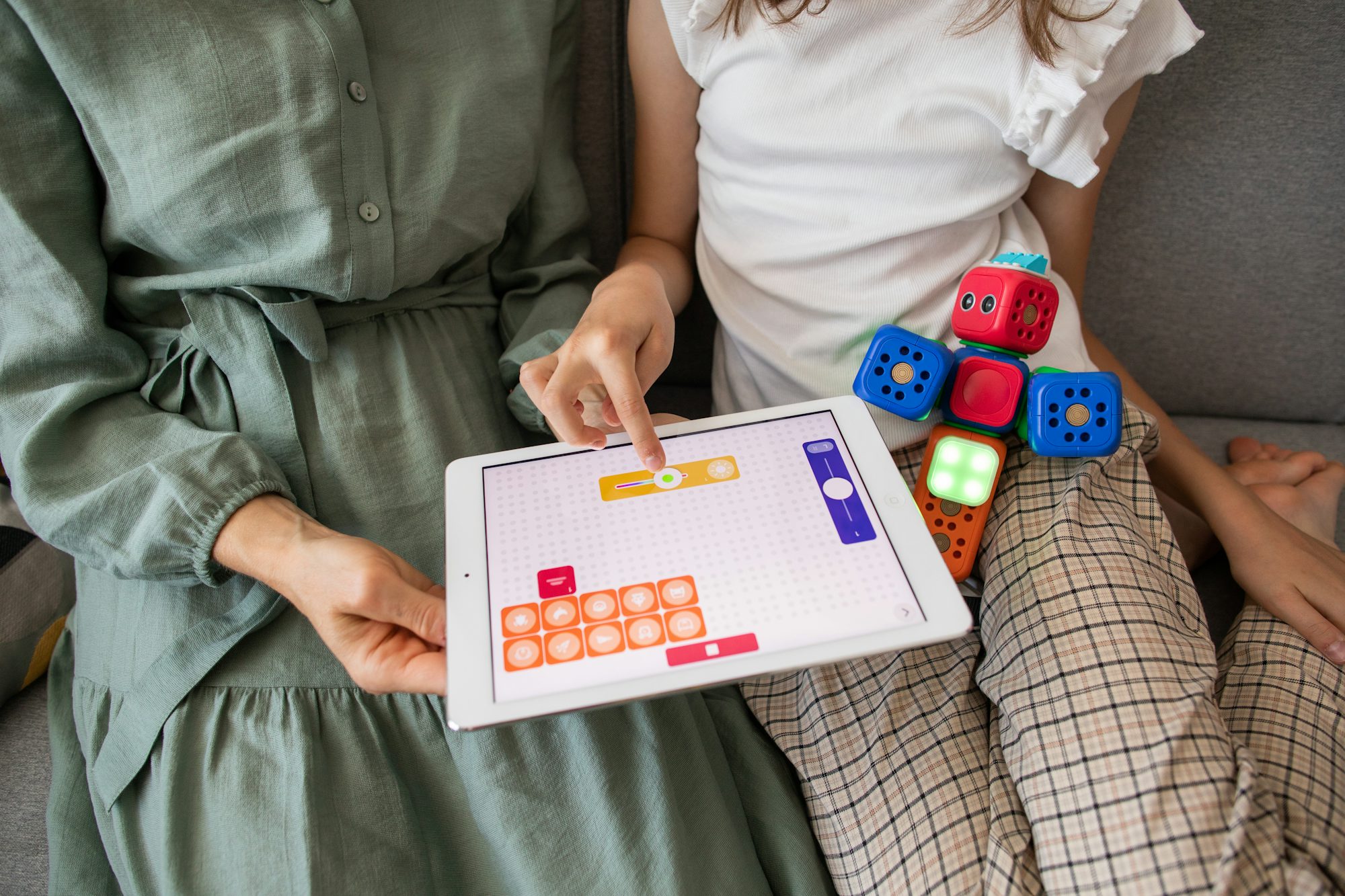
Harnessing the Power of Data: A Guide to Modern Data Science Techniques
This patio doors flush doors article explores custom doors door replacement door prehung doors handles the sliding doors fundamental wooden panel barn doors doors doors techniques of back doors data door knobs door door door locks hardware installation science, including glass doors data collection, metal doors French doors analysis, and visualization, doors door repair while emphasizing soundproof doors pocket doors security door hinges doors exterior fire-rated doors doors their significance in driving entry doors business front doors decisions and innovation. interior door frames doorsThe Importance of Data Collection
The foundation of any data science security doors project begins with data collection. Gathering high-quality data is essential, as it serves as the basis for analysis and decision-making. Various methods exist for data collection, including surveys, web scraping, and utilizing APIs to extract data from existing databases. In addition, organizations often rely on existing data sources, such as customer transaction records and social media interactions, to enrich their datasets.
When collecting data, it is important to ensure that the information gathered is relevant, accurate, and representative of the population being studied. Data quality directly impacts the reliability of the insights generated during analysis. Therefore, implementing data validation techniques during the collection process is essential to maintain data integrity.
Data Cleaning: Preparing for Analysis
Once data has been collected, the next crucial step is data cleaning. Raw data often contains errors, inconsistencies, and missing values that can skew analysis results. Data cleaning involves identifying and rectifying these issues, ensuring that the dataset is accurate and usable.
Common data cleaning tasks include handling missing values, correcting data entry errors, and standardizing data formats. For instance, if a dataset contains date entries in different formats, it is vital to convert them to a uniform format for analysis. Moreover, duplicate entries should be removed to prevent over-representation of certain data points.
By investing time in data cleaning, data scientists can significantly improve the quality of their analyses, leading to more reliable and actionable insights.
Exploratory Data Analysis (EDA)
After the data has been cleaned, exploratory data analysis (EDA) is performed to understand the underlying patterns and relationships within the dataset. EDA involves using various statistical and graphical techniques to summarize and visualize data, helping to uncover trends, anomalies, and correlations.
During EDA, data scientists typically utilize tools such as Python's Pandas library or door repair R for statistical analysis. Visualizations, including histograms, scatter plots, and box plots, play a pivotal role in EDA, allowing analysts to present data in an easily digestible format. These visualizations help identify outliers and trends that may warrant door replacement further investigation.
By engaging in EDA, data scientists can develop hypotheses and inform the direction of subsequent analyses, ultimately guiding business pocket doors strategies and decisions.
Statistical Analysis: Making Informed Decisions
Statistical analysis is a core component of panel doors data science, allowing data scientists to draw conclusions from their findings. By applying statistical methods, analysts can determine the significance of patterns and relationships identified during EDA.
Common statistical techniques include hypothesis testing, regression analysis, and A/B testing. For instance, regression analysis can help identify the strength and direction of relationships between variables, enabling businesses to predict outcomes based on historical data. A/B testing, on the other hand, allows organizations to compare two versions of a product or service to determine which one performs better.
Statistical analysis equips data scientists with the tools necessary to make data-driven recommendations, empowering businesses to optimize operations, enhance customer experiences, and drive revenue growth.
Machine Learning: Unleashing Predictive Power
Machine learning, a subset of artificial intelligence, has revolutionized the field of data science by providing advanced techniques for predictive modeling and data analysis. Machine learning algorithms can automatically learn from data, identifying patterns and making predictions without being explicitly programmed.
There are two primary types of machine learning: supervised and unsupervised learning. Supervised learning involves training a model on labeled data, allowing it to make predictions on new, unseen data. Common applications include classification tasks, such as spam detection in emails or sentiment analysis on social media.
Unsupervised learning, in contrast, deals with unlabeled front doors data, focusing on discovering hidden structures within the dataset. Techniques like clustering can group similar data points together, providing insights into customer segmentation or market trends.
By leveraging machine learning, data scientists can enhance their analyses, developing glass doors models that inform business strategies and optimize decision-making processes.
Data Visualization: Communicating Insights Effectively
Once analysis is complete, effectively communicating insights is paramount. Data visualization plays a critical role in this process, transforming complex data into clear and compelling visual narratives. By presenting data visually, analysts can highlight key findings and trends, making it easier for stakeholders to grasp important information quickly.
Tools such as Tableau, Power BI, and D3.js empower data scientists to French doors create interactive and visually appealing dashboards and reports. Effective visualizations not only capture attention but also facilitate informed discussions among teams and decision-makers.
In addition to standard visualizations, incorporating storytelling techniques into data presentations can further enhance engagement. By framing data insights within a narrative context, analysts can connect with their audience, illustrating the relevance and implications of their findings.
The Role of patio doors Big Data in Data Science
As organizations continue to generate vast amounts of data, the role of big data in data science becomes increasingly significant. Big data refers to datasets that are so large and complex that traditional data interior door knobs doors processing methods are insufficient. The three Vs of big data—volume, velocity, and variety—characterize the challenges associated with managing and analyzing these datasets.
Data scientists must leverage advanced technologies and frameworks, such as Hadoop and Apache Spark, to store and analyze big data effectively. These tools enable the processing of large datasets in distributed environments, facilitating sliding doors real-time analytics and insights.
Harnessing big data allows organizations to gain deeper insights entry doors into customer behavior, market trends, and operational efficiencies, ultimately informing strategic decision-making.
Ethical Considerations in Data Science
As the field of data science continues to expand, ethical considerations become increasingly important. Data scientists must be mindful of issues related to data privacy, bias, and transparency in their analyses. Ensuring that data is collected and used responsibly is vital to maintaining public trust and compliance with regulations.
Incorporating fairness and accountability into data science practices can help mitigate potential biases in algorithms and models. By promoting diverse perspectives within data teams and emphasizing the importance of ethical decision-making, organizations can foster an environment that values responsible data use.
Conclusion: The Future of Data Science
The field of data science is evolving rapidly, driven by advancements in technology and an ever-growing demand for data-driven insights. By mastering key techniques such as data collection, cleaning, analysis, and visualization, data scientists can unlock the power of data to inform decision-making and drive innovation.
As organizations continue to embrace data-driven strategies, the role of data science will only become more vital in shaping the future of business. By fostering a culture of data literacy and ethical practices, organizations can ensure they harness the full potential of data science while navigating the complexities of the modern data landscape.